|
ACCESS THE FULL ARTICLE
No SPIE Account? Create one
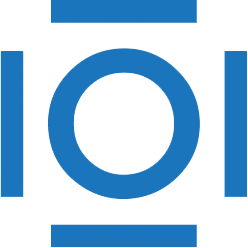
CITATIONS
Cited by 3 scholarly publications.
Artificial neural networks
Image classification
Data modeling
Neurons
Satellites
Matrices
Modeling