|
ACCESS THE FULL ARTICLE
No SPIE Account? Create one
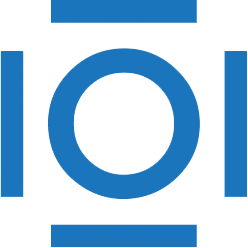
CITATIONS
Cited by 2 scholarly publications.
Sensors
General packet radio service
Digital filtering
Signal processing
Monte Carlo methods
Statistical analysis
Antennas