|
ACCESS THE FULL ARTICLE
No SPIE Account? Create one
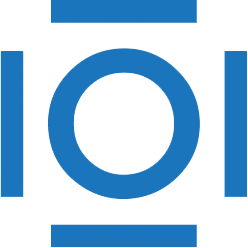
CITATIONS
Cited by 3 scholarly publications.
Signal processing
Data modeling
Reliability
Stochastic processes
Atrial fibrillation
Autoregressive models
Deconvolution