|
ACCESS THE FULL ARTICLE
No SPIE Account? Create one
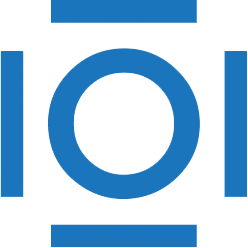
CITATIONS
Cited by 1 scholarly publication.
Wavelets
Data modeling
Functional magnetic resonance imaging
Data analysis
Smoothing
Modeling
Neuroimaging