|
ACCESS THE FULL ARTICLE
No SPIE Account? Create one
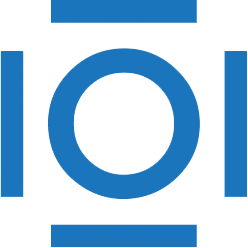
CITATIONS
Cited by 1 scholarly publication and 3 patents.
Databases
Image resolution
Image scaling
Image analysis
Image processing
Spatial analysis
Detection and tracking algorithms