|
ACCESS THE FULL ARTICLE
No SPIE Account? Create one
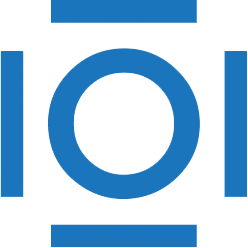
CITATIONS
Cited by 2 scholarly publications.
Digital signal processing
Seaborgium
Data modeling
Mining
Pattern recognition
Data mining
Information science