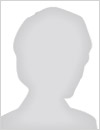
Major calcifications are of great concern when performing percutaneous coronary interventions because they inhibit proper stent deployment. We created a comprehensive software to segment calcifications in intravascular optical coherence tomography (IVOCT) images and to calculate their impact using the stent-deployment calcification score, as reported by Fujino et al. We segmented the vascular lumen and calcifications using the pretrained SegNet, convolutional neural network, which was refined for our task. We cleaned segmentation results using conditional random field processing. We evaluated the method on manually annotated IVOCT volumes of interest (VOIs) without lesions and with calcifications, lipidous, or mixed lesions. The dataset included 48 VOIs taken from 34 clinical pullbacks, giving a total of 2640
We created and evaluated a processing method for dynamic computed tomography myocardial perfusion imaging (CT-MPI) of myocardial blood flow (MBF), which combines a modified simple linear iterative clustering algorithm (SLIC) with robust perfusion quantification, hence the name SLICR. SLICR adaptively segments the myocardium into nonuniform super-voxels with similar perfusion time attenuation curves (TACs). Within each super-voxel, an α-trimmed-median TAC was computed to robustly represent the super-voxel and a robust physiological model (RPM) was implemented to semi-analytically estimate MBF. SLICR processing was compared with another voxel-wise MBF preprocessing approach, which included a spatiotemporal bilateral filter (STBF) for noise reduction prior to perfusion quantification. Image data from a digital CT-MPI phantom and a porcine ischemia model were evaluated. SLICR was ∼50-fold faster than voxel-wise RPM and other model-based methods while retaining sufficient resolution to show clinically relevant features, such as a transmural perfusion gradient. SLICR showed markedly improved accuracy and precision, as compared with other methods. At a simulated MBF of 100 mL/min-100 g and a tube current–time product of 100 mAs (50% of nominal), the MBF estimates were 101 ± 12, 94 ± 56, and 54 ± 24 mL / min-100 g for SLICR, the voxel-wise Johnson–Wilson model, and a singular value decomposition-model independent method with STBF, respectively. SLICR estimated MBF precisely and accurately (103 ± 23 mL / min-100 g) at 25% nominal dose, while other methods resulted in larger errors. With the porcine model, the SLICR results were consistent with the induced ischemia. SLICR simultaneously accelerated and improved the quality of quantitative perfusion processing without compromising clinically relevant distributions of perfusion characteristics.
We developed machine learning methods to identify fibrolipidic and fibrocalcific A-lines in intravascular optical coherence tomography (IVOCT) images using a comprehensive set of handcrafted features. We incorporated features developed in previous studies (e.g., optical attenuation and A-line peaks). In addition, we included vascular lumen morphology and three-dimensional (3-D) digital edge and texture features. Classification methods were developed using expansive datasets (∼7000 images), consisting of both clinical
Our results indicate that when implementing the process on a stationary acquisition dataset, the uncertainty improves at each stage while the uncertainty is reduced. When comparing stationary acquisition dataset to pullback dataset, the values were as follows: calcium: 3.8±1.09mm-1 in stationary and 3.9±1.2 mm-1 in a pullback; lipid: 11.025±0.417 mm-1 in stationary and 11.27±0.25 mm-1 in pullback; fibrous: 6.08±1.337 mm-1 in stationary and 5.58±2.0 mm-1. These results indicates that the process presented in this paper introduce minimal bias and only a small change in uncertainty when comparing a stationary and pullback dataset, thus paves the way to a highly accurate clinical plaque type discrimination, enabling automatic classification.
View contact details