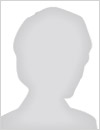
SHARK-NIR is an instrument which provides direct imaging, coronagraphic imaging, dual band imaging and low resolution spectroscopy in Y, J and H bands, taking advantage of the outstanding performance of the Large Binocular Telescope AO systems. Binocular observations will be provided used in combination with SHARK-VIS (operating in V band) and LMIRCam of LBTI (operating from K to M bands), in a way to exploit coronagraphic simultaneous observations in three different wavelengths.
A wide variety of coronagraphic techniques have been implemented in SHARK-NIR, ranging from conventional ones such as the Gaussian Lyot, to others quite robust to misalignments such as the Shaped Pupil, to eventually techniques more demanding in term of stability during the observation, as the Four Quadrant; the latter is giving in theory and simulations outstanding contrast, and it is supported in term of stability by the SHARK-NIR internal fast tip-tilt loop and local NCPA correction, which should ensure the necessary stability allowing this technique to operate at its best.
The main science case is of course exoplanets search and characterization and young stellar systems, jets and disks characterization, although the LBT AO extreme performance, allowing to reach excellent correction even at very faint magnitudes, may open to science previously difficult to be achieved, as for example AGN and QSO morphological studies.
The institutes participating to the SHARK-NIR consortium which designed and built the instrument are Istituto Nazionale di Astro Fisica (INAF, Italy), the Max Planck Institute for Astronomy (MPIA, Heidelberg, Germany) and University of Arizona/Steward Observatory (UoA/SO, Tucson, Az, USA). We report here about the SHARK-NIR status, that should achieve first light at LBT before the end of 2022.We investigate the adoption of Machine Learning techniques for piston sensing in the context of segmented primary mirror telescopes by the means of numerical simulations. Considering a Natural Guide Star Wavefront Sensor, composed by one high order modes sensor plus a second sensor dedicated to the differential piston modes, we focus on the latter and tackle the problem of providing an accurate estimation for the piston modes coefficients from a defocused image of the system PSF.
We consider as a baseline algorithm a customized version of LIFT (which is based on a Maximum Likelihood Estimation) and compare its performance with a Deep Neural Network (DNN) regression. After considering several DNN architectures, we designed a simple one and performed some degree of hyperparameter optimization on it to obtain the final DNN version.
The code we developed is written in Python and relies on the Tensorflow4 library and its numerical backend JAX3.View contact details